Responsible AI Governance: Beyond the ‘Department of No’
AI
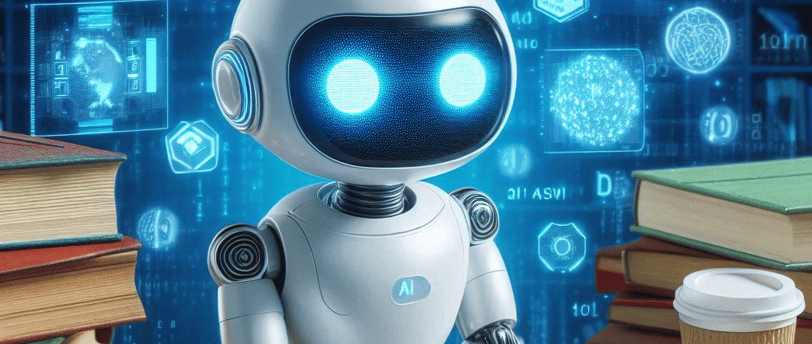
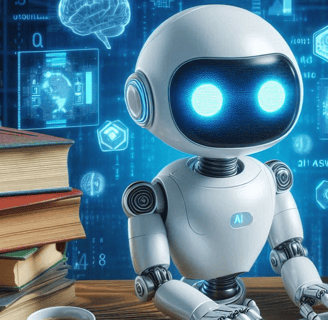
For organisations at the beginning of their AI governance journey, it can be intimidating. AI technology is advancing rapidly, with GPT-5 expected to be released later this year. While the UK government whitepaper 'A-pro innovation approach to AI regulation' provides non-statutory ethical principles, there is uncertainty about whether the UK will move forward with a statutory approach like the EU AI Act. At present, this seems unlikely.
In absence of cross-cutting AI regulation, how can organisations create future-proof governance policies and practices? In particular, how do they operationalise the whitepaper principles?
Guidance from organisations like the Responsible Technology Adoption (RTA) unit, and emerging standards for AI governance such as ISO 42001 are beginning to plug the gap, but there is still work to be done to translate this into internal governance.
This is especially challenging when innovation and safety are perceived as a tradeoff. The risk of adopting AI too early and getting things wrong has a high cost, especially in the public sector where public trust is paramount. Equally, adopting too late carries a steepening opportunity cost. How do public sector organisations start to reconcile this? How do they start to govern AI effectively without becoming perceived as the 'department of no'?
In this article, I share my personal thoughts as a data professional on this issue inspired by my research into AI Ethics and Responsible AI, and engagement with industry, academia and government organisations to "compare notes" on approaching the issue. While this is inspired by my formative experience developing a Responsible AI governance strategy in a public sector organisation in the UK, please note that this article represents my personal views and not the views of my employer or any other specific organisation.
Go back to basics.
In order to ground myself, I have found it helpful to remember that AI starts with data, and that garbage in = garbage out (GIGO) when it comes to AI models. Robust data governance underpins robust AI governance.
The ‘Use of Artificial Intelligence in Government’ report by the National Audit Office identified access to good-quality data as a barrier to implementing AI by the majority of government bodies surveyed. When approaching AI, don’t neglect revisiting the data maturity of the organisation, including the evaluation of existing data condition and data management practices. Reflect on how the organisation approached readiness to GDPR and the lessons learnt. Going back to basics will provide a strong basis to take confidence from when addressing AI risk.
Own the risk.
It is wise to be proactive in creating ownership for AI risk within the organisation as early as possible. This may initially start with the creation of a dedicated research group to evaluate AI risk.
A logical next step would be creating an accountability for AI Risk & Governance within the organisation. Assigning a reporting line where the accountable individual(s) for AI governance sits can be a difficult decision with tradeoffs in terms of suitability. For example, while the Chief Technology Officer (CTO) is well positioned due to their understanding of AI technology, they may not be sufficiently independent enough to own AI risk. Should the accountability for leading the AI technology adoption strategy be independent from the accountability for compliance/risk management? If these accountabilities are centralised, this could lead to a conflict of interest where the CTO ends up “marking their own homework”.
It may be worthwhile to pool the risk by creating a joint accountability for AI governance (for example, the Chief Data Officer together with the Chief Security Officer) or creating an AI steering group or Ethics Board with a broader range of C-Level represented. There is no perfect answer here; be proactive and take a decision early to begin addressing AI risk, while recognising that even after assigning accountability, a multidisciplinary approach will still be required.
Don't wait for perfect. Build the foundations.
Uncertainty about the acceleration of AI technology and how regulators will respond can make it tempting to delay AI governance. There is a worry that imperfect governance policies will quickly become redundant. This is underscored by fears that governance will come in the way of early adoption of AI. A good way to navigate this is by developing something foundational and iterating. Focus on laying the groundwork for AI governance, while operating within a strict timebox and narrowing the scope accordingly. Consider what governance practices can be implemented within, say, the next six months.
It may be worthwhile to prioritise operationalising a specific principle, such as transparency: setting a goal to simply establish oversight of AI use within the organisation by creating a register of use cases. The Algorithmic Transparency Recording Standard (ATRS) guidance is a good place to start.
It may even be beneficial to narrow the scope to a specific technology, for example, public generative AI web services: setting a goal to publish guardrails for employee use of public LLMs like ChatGPT. The Generative AI Framework for HM Government provides a good starting point for this. Rather than having a fixed schedule for updates, create an accountability for refreshing and adapting this guidance in response to milestone tech/regulatory changes.
Reframe. Think about what you can say yes to.
Fears about the existential risk of AI can distract from the reality that there will likely be many existing lower-risk use cases for AI and ML technology within the organisation. Start having honest conversations with C-Level about the business risk appetite for AI. Work with data scientists and analysts to define lower-risk use cases for the organisation, and continue to move forward with these.
Don't reinvent the wheel.
Lean on existing governance processes rather than starting from scratch. AI does carry unique structural risks as compared with other technologies, and so existing governance practices may not be entirely fit-for-purpose to achieve Responsible AI outcomes. Acknowledging this, there will still be existing governance that can be used as a foundation for AI governance, or at least aligned to.
A good place to start would be to reflect on the existing values of the organisation (or more specifically, data ethics principles if these exist), and how these can be aligned to the whitepaper principles. Consider which existing governance structures could be adapted to support AI governance (e.g. an existing ethics board). Model existing governance processes and consider where the touchpoints or escalation channels could be for ethical AI evaluation.
Collaborate. Create the building blocks and iterate.
The most critical driver to AI adoption is trust. As you move forward with lower-risk use cases for AI, use these to test out developing governance practices. Document the lessons learnt to build up a knowledge bank of what governance practices are most effective. This will help to build trust and confidence when it comes to evaluating and deploying higher-risk use cases.
Work collaboratively on this - get something out there, get feedback on it and evolve. The structural risks of AI are multidisciplinary, so developing AI governance demands a collaborative approach. Start building relationships across the organisation, and invite feedback on any governance proposals as early as possible. Forget about "IT" and "The Business" - Responsible AI is not just a tech/data issue.
Legal Services can be a great area to start with: for AI to be ethical, it must at the minimum be legal. Start having conversations about how information rights law is evolving in the age of AI, and what areas will be key to consider in any governance framework. Work with cyber security experts to address resilience and robustness of AI systems. Speak to R&D teams about how to leverage academic research in Responsible AI. Speak to environmental teams about how to address the resource cost of training compute and run-time of AI models. Speak to commercial teams about how to update procurement processes and align internal governance standards with those of the supply chain. Speak to HR about this in the context of the recruitment sector; the RTA’s recent Responsible AI in Recruitment guide is a good place to start for framing these conversations.
While taking a collaborative approach can take time, it will lead to more sustainable AI governance through generating better insights and greater buy-in to Responsible AI within the organisation.
Go beyond governance: Responsible AI is a culture shift.
Build a narrative about what Responsible AI means and why it matters. Start by making sure everyone is on the same page about what AI is and how the organisation needs to adapt to manage it. Set the scene with an educational awareness campaign about AI and the risks and opportunities it presents.
Highlight case studies where governance practices resulted in effective risk-mitigation and led to better outcomes. Equally, don't shy away from elevating examples where poor governance led to negative outcomes, and the reputational consequences of this. For example, ICO’s enforcement action against Serco for using facial recognition tech for attendance monitoring (this was deemed an unlawful processing of biometric data) brings this to life.This is not about scaremongering, but about having honest conversations about the reality of unintended consequences of poorly governed AI.
Creating this narrative, together with fostering a collaborative approach, will help to drive buy-in to AI governance. More importantly, it will create the culture shift needed to implement Responsible AI.
These are my personal thoughts on AI governance, and I welcome any insights and opportunities for further discussion.
References:
A pro-innovation approach to AI regulation, Department for Science, Innovation and Technology (DSIT)
Generative AI Framework for HM Government, Central Digital and Data Office (CDDO)
Algorithmic Transparency Recording Standard, CDDO & Responsible Technology Adoption (RTA) Unit
Responsible AI in Recruitment, RTA Unit
ISO 42001, British Standards Institute (BSI) Group
Use of artificial intelligence in government, DSIT, National Audit Office